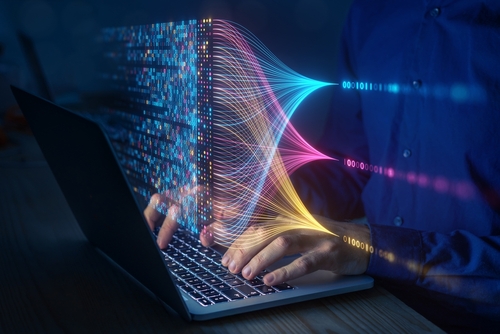
How Eniac invests in AI at the application layer
By Hadley Harris and Coleman Clyde
AI is obviously the hottest startup sector right now, but also the most overhyped — that was the overwhelming message from investors who participated in Eniac’s Q2 Seed Sentiment Survey. So what kind of framework can seed firms like Eniac use to invest in AI?
To be clear, we aren’t starting from scratch. Far from it: Our investments in the sector go back at least a decade and over the past few years we’ve backed exciting AI startups like Attention, Fabi.ai, Bito.ai, LevelAI, Pienso, Sutro, Unsupervised, Approximate Labs and Kindo AI.
Still, given both the tremendous potential of AI and the speed with which the field is evolving, we wanted to dive deeper and ensure that we’re making the smartest bets we can, with an initial focus on the application layer — where startups are often dismissed as little more than a “thin wrapper” for platforms like ChatGPT. Inspired by our friend Aubrie Pagano’s “field studies” approach, we decided to spend some time reading widely and talking to some of the smartest people we know (founders, operators, and subject matter experts) to answer the following questions:
What should Eniac’s investment framework be for investing in AI at the application layer? What are the attributes we want and don’t want to see in generative AI application investments?
Of course, we already had some thoughts about how to answer these questions, based on our years of investing in AI and the countless conversations we’d already had with experts. But this research project allowed us to synthesize and hone those thoughts into the following framework:
Key attributes that we look for:
- Pain killer products with robust scaffolding
The capabilities of LLMs and other generative AI models have significantly reduced the barriers to creating useful, previously unseen tools for end users. As a result of heightened competition and rapid innovation in the space, it’s increasingly important to seek out companies that are building on the core principles of creating durable software solutions. These principles include offering a product or service that solves significant user pain points, delivering a seamless user experience, providing straightforward integrations with existing data and software, and establishing robust workflows around the core product. These characteristics help differentiate AI applications from foundational models like ChatGPT, and create switching costs for B2B customers.
While a useful AI-powered tool can be an effective wedge into a new market, the scaffolding and workflows built around that product will likely determine its defensibility over time.
2. Founding teams with deep domain expertise
Most generative AI applications likely won’t be able to differentiate on their tech stack. As a result, we believe that deep domain expertise will be critical in developing a competitive moat and differentiated offering.
Domain experts intimately understand the problems their customers are facing, as well as the solutions they’re currently using. This is particularly important when deploying a new technology that’s rapidly evolving. Domain experts are better-positioned to know how to implement generative AI-powered tools in a way that’s tailored to the needs of their customer, resulting in better products and a more efficient sales process.
3. Vertical offerings
Similar to domain expertise, vertical AI solutions create an opportunity to gain a competitive advantage by leveraging industry-specific expertise and data to build superior products. Industry expertise helps founders better understand the problems facing their end customer and where LLMs can add value to critical workflows. While the benefits of using proprietary data to fine tune a baseline model are debated, industry-specific offerings arguably will have access to some of the most robust and unique data sets. These applications can also benefit from similar advantages enjoyed by vertical SaaS players (winner takes most market dynamics, clear and targeted value prop, lower CAC, higher customer retention).
4. Flexible teams with the ability to rapidly iterate on customer feedback
Given the high level of uncertainty around generative AI best practices, we believe a flexible founding team with a willingness to rapidly iterate on how they’re deploying technology, paired with frequent feedback loops from customers, can be a competitive advantage over the medium term. While we look for this across all our investments, this is especially important for generative AI applications given the pace of innovation, which creates a need for more frequent feedback loops.
While creating a sustainable competitive advantage on the technology itself is an uphill battle, CTOs who are flexible and willing to iterate creatively will have the best chance of staying at the head of the pack in a rapidly-evolving environment. This might include building a product with the ability to switch between different foundational models to optimize for use case, speed, and cost. It also might include the creative implementation of LLMs through prompt engineering, model chaining, fine tuning of open sourced models, etc. Early results show that minor tweaks to prompting and model implementation can vastly improve output, highlighting the value of creativity and rapid iteration.
The ability to access frequent customer feedback data via tight communication with clients will also better-position startups to iterate faster than their competitors. While always important for B2B applications, this is particularly important for generative AI-powered applications.
5. Startups competing with vulnerable legacy incumbents
The answer to “startup vs incumbent” in the generative AI application arms race varies widely by the characteristics of the incumbents themselves. In our view, incumbents with the following characteristics offer the greatest opportunity for disruption:
- Outdated technology — The most obvious markets for gen-AI startups to attack are legacy players who make limited use of technology in their existing product/service. This might include service-based businesses that have historically relied on humans (e.g. lawyers or consultants), or industries with low tech adoption rates (construction, education, healthcare, etc.).
- Resistance to change — Incumbents with a low sense of urgency to adapt are also vulnerable. These firms are often deeply rooted in older, legacy systems and methodologies, and tend to be risk-averse, making them slow to implement changes to their technology and business model.
- Innovator’s dilemma — While some incumbents possess the technological capabilities and resources to implement AI-based solutions, they may be resistant to do so for fear of cannibalizing their existing business models. Google is a great example of this type of incumbent, as the rapid deployment of chat-based search engines could disrupt its most important business line (ads).
Common traits we avoid:
- Applications that consider an undifferentiated tech stack to be a competitive advantage
New startups with truly unique technology stacks at the application layer are very rare, given the technical and financial resources needed to compete with well-funded players such as OpenAI and Anthropic. Far more common are startups who believe to have built a defensible company based on an undifferentiated application of the baseline technology. These founders will often overlook the traits that can result in a true long-term moat (scaffolding, domain expertise, feedback loops, etc.).
2. Shiny hammers without a nail
The potential use cases for generative AI are seemingly limitless, with new tools being developed almost daily. However, many of these tools are being built because they can be, not because there is a real need for them. In most cases, we would shy away from investing in founders who are overly focused on what their product can do, instead of what problem it solves. Founders building shiny hammers often lack domain expertise, and therefore fail to deeply understand the needs of their customers and how to build scaffolding around their baseline application.
3. ChatGPT wrapper tools without a long-term plan
Applications that appear different from ChatGPT on the surface — a unique UX/UI, a few added features, a targeted use case — but generate similar outcomes will likely lack defensibility over the long term. We are wary of products whose main value-add is to produce content, as they are vulnerable to copycats and may be easily replicated using prompt engineering with an existing foundational model (like ChatGPT).
Exceptions to this rule are founders who are thoughtfully using a wrapper tool as a way to quickly go to market, but who have a clear plan for how they will build scaffolding around their MVP to make it a durable business over time.
4. Low volume, high risk use cases
Startups solving problems that require highly-accurate solutions with a limited volume of data to train on will likely be slow to scale given LLMs still struggle with hallucination. In general, applications with use cases that aren’t useful without major improvements being made in the accuracy and efficacy of existing LLMs would give us pause.
Disputed point:
Use of proprietary data as a competitive moat
Many view access to proprietary datasets that can be used to fine tune the foundational model as a potential competitive moat. Specialized datasets could in theory improve the output of the model in a differentiated way, though this is yet to be proven out. Those who oppose this viewpoint believe that outcomes achieved by training an LLM on specialized datasets won’t be differentiated from what foundational models will be able to achieve through few shot prompting (providing explicit examples to guide the model’s response). They are also skeptical of the durability of “proprietary data” given a shifting trend toward open source models. It is unclear whether fine tuning a model will achieve superior results to prompt engineering.
Think you’ve got a startup that meets these criteria? Please reach out!